A recent study published in Computers in Biology and Medicine the world’s second-ranked journal in its field, introduces a groundbreaking AI system to predict cell migration in breast cancer. The study, led by researchers from the University of Granada and the University of Seville, presents significant advancements in the use of deep learning and computational biology for cancer research.
Leveraging Deep Learning for Breast Cancer Research
The research team, which includes Francisco M. García-Moreno, a member of the MYDASS Research Group and of the OnTheEdge R&D Project, as well as PhD candidate Jesús Ruiz Espigares, Juan Antonio Marchal Corrales, and Miguel Ángel Gutiérrez Naranjo, developed the Prediction Wound Progression Framework (PWPF). This framework utilizes deep learning techniques to predict the dynamic evolution of cell migration in Wound Healing Assays, offering new insights into the progression of breast cancer metastasis.
Wound Healing Assays are a standard method for studying cell movement in two-dimensional models, providing essential data for understanding how cell migration impacts the metastasis process. By accurately predicting the movement of cancer cells, the PWPF framework contributes to improving our knowledge of how metastasis occurs, leading to potential advancements in therapeutic strategies.
Predicting Cell Migration with AI: A Technological Breakthrough
The team used a Conv-LSTM neural network architecture, which is specifically designed to capture both spatial and temporal data from Wound Healing Assays. This unique approach allows researchers to predict how cancer cells will migrate over time, significantly enhancing the ability to study breast cancer dynamics. While the framework currently focuses on two-dimensional models, it shows great promise for application in 3D models that can more accurately mimic real tumor behavior.
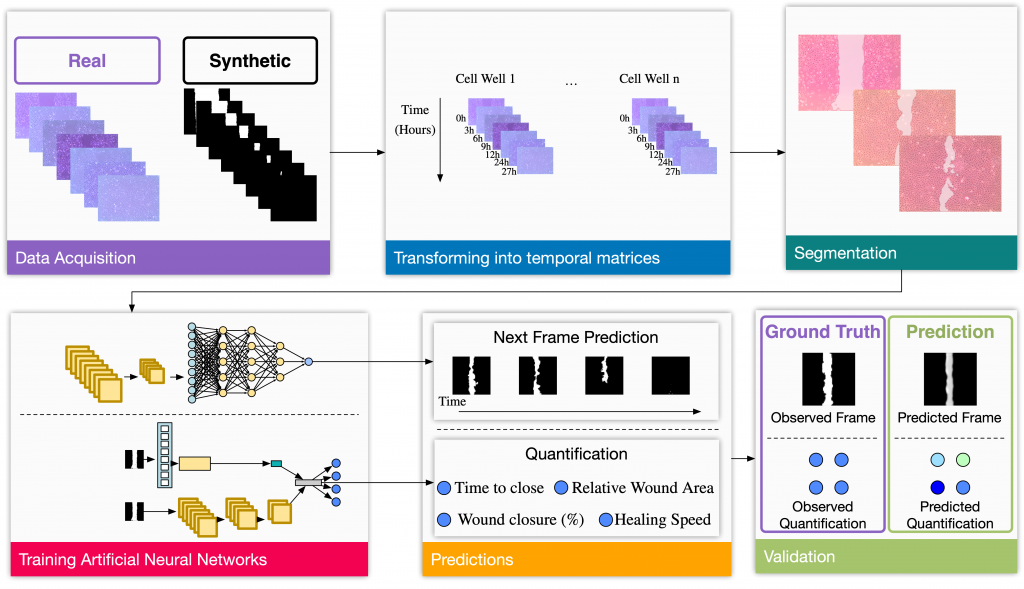
“Metastasis is the leading cause of mortality in breast cancer patients, and understanding how cell migration occurs is essential for developing more effective treatment strategies,” explains Jesús Ruiz, co-principal investigator from the Department of Human Anatomy and Embryology at the University of Granada.
A Multidisciplinary Collaboration
This research is the result of a collaborative effort between multiple departments and research centers, including the Department of Computer Languages and Systems (LSI), the Department of Human Anatomy and Embryology, and CITIC at the University of Granada. Additionally, the project involves the BioFabi3D_Biofabrication and 3D (Bio)printing Lab at CIBM, the Unit of Excellence “Modeling Nature,” and the Institute for Biosanitary Research ibs.GRANADA, with support from the University of Seville’s Department of Computer Science and Artificial Intelligence.
The open access nature of the research is one of its most notable features. The code and data generated by the team are available on GitHub and Zenodo, encouraging further collaboration in the global fight against cancer.
Impact and Future Directions
The project’s findings have the potential to significantly improve the understanding of breast cancer metastasis and may open new pathways for both research and treatment. By applying the Prediction Wound Progression Framework to more complex models, such as 3D tumor models, researchers can explore how different treatments may influence cell migration and metastasis in a more realistic environment.
Funded by the Ministry of Science, Innovation, and Universities (MICIN), the Ministry of Health and Families of the Government of Andalusia, and the Doctors Galera and Requena Chair of Cancer Stem Cell Research at the University of Granada, this project exemplifies the importance of multidisciplinary, collaborative research in advancing the field of breast cancer treatment.
Reference
Garcia-Moreno FM, Ruiz-Espigares J, Gutiérrez-Naranjo MA, Marchal JA. Using deep learning for predicting the dynamic evolution of breast cancer migration. Comput Biol Med. 2024 Sep;180:108890.